Labeling Anomaly Detection flags potential errors in document annotations, helping train more accurate models, faster.
On March 21st, Hyperscience launched Labeling Anomaly Detection, an AI-assisted way to flag anomalies in annotation and generate accurate ground truth data. Back in December we launched Guided Data Labeling which provided customers with a faster, smoother experience for gathering and annotating training data.
Customers can leverage both of these features to get ML assistance when annotating data (Guided Data Labeling), and to fix any anomalies in this annotation (Labeling Anomaly Detection). These features work hand-in-hand to reduce time-to-value by allowing customers to train their machine learning models faster—and a fine-tuned model based on accurate company data will always lead to better end results.
Why use Labeling Anomaly Detection?
When implementing machine learning models, the time needed to train accurate models can delay the full-scale rollout of the solution. It’s a conundrum often faced—do you go live, knowing there might be some inaccuracies in your “ground truth” data, or do you spend extra time and resources getting the source data as water-tight as possible, delaying the launch of a transformative investment?
The problem is compounded with every layout variation that is added to a use case. Additionally, going back to the source to find the errors in the “ground truth” requires hours spent retraining and performing multi-variant testing to isolate the issue.
Labeling Anomaly Detection provides the best of both worlds—accurate ground truth and faster training times.
After documents have been annotated, this new feature flags potential errors in the annotations. You can then review these potential anomalies and either mark them as correct or make any corrections—ensuring your data is as accurate as possible out of the gate.
Key Benefits of Labeling Anomaly Detection
By detecting anomalies before they’re used for model training, the resulting model can be trained more quickly, and the end result is more accurate. A machine learning model that can flag possible annotation anomalies provides several benefits. Here are just three ways that Labeling Anomaly Detection provides value to organizations.
Improved Accuracy and Reliability
Labeling Anomaly Detection helps improve the accuracy and reliability of annotated data by identifying and highlighting any potential errors or inconsistencies. This, in turn, leads to better and more reliable results when using the annotated data for training machine learning models or conducting other data analysis tasks.
Saved Time and Resources
Labeling Anomaly Detection also saves time and human resources by automating the process of identifying annotation anomalies, which can be a time-consuming and tedious task for human annotators.
Downstream Error Prevention
By detecting anomalies and correcting annotation errors early on, Labeling Anomaly Detection helps prevent downstream errors and biases that can arise when using flawed annotated data.
How Labeling Anomaly Detection works
Any user can use Labeling Anomaly Detection on their annotated data by simply running analysis on their identification model ground truth in the Keyer Data Management screen. The following steps illustrate how to use Guided Data Labeling and Labeling Anomaly Detection to dramatically improve the performance of semi-structured identification models.
Step 1: Upload documents
Upload the required number of training documents into Keyer Data Management.
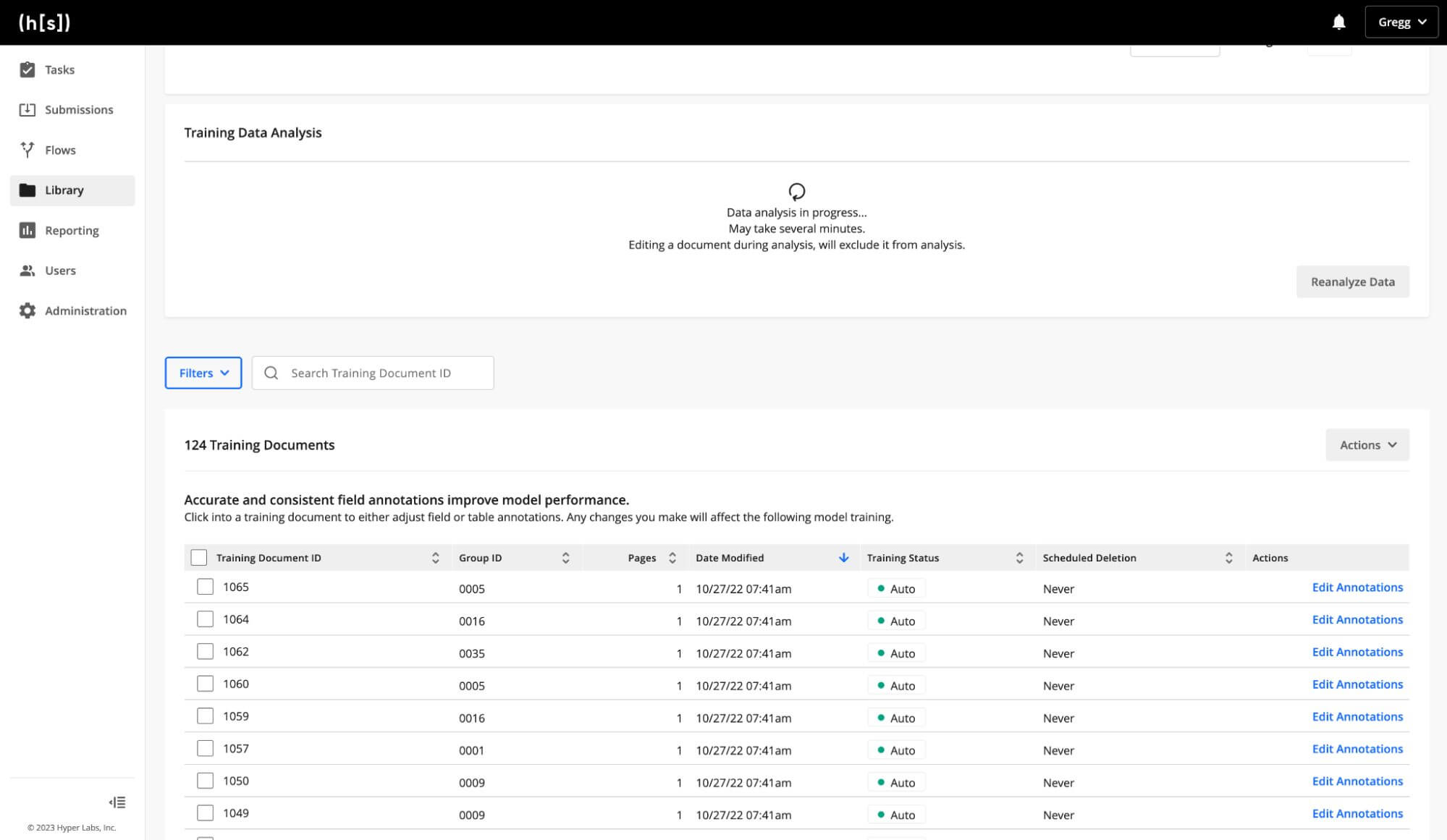
Step 2: Analyze the data
Run ‘Analyze Data,’ found in the Training Data Analysis card for your semi-structured identification model. When analysis is complete, your training documents will be organized in clusters of similar documents.
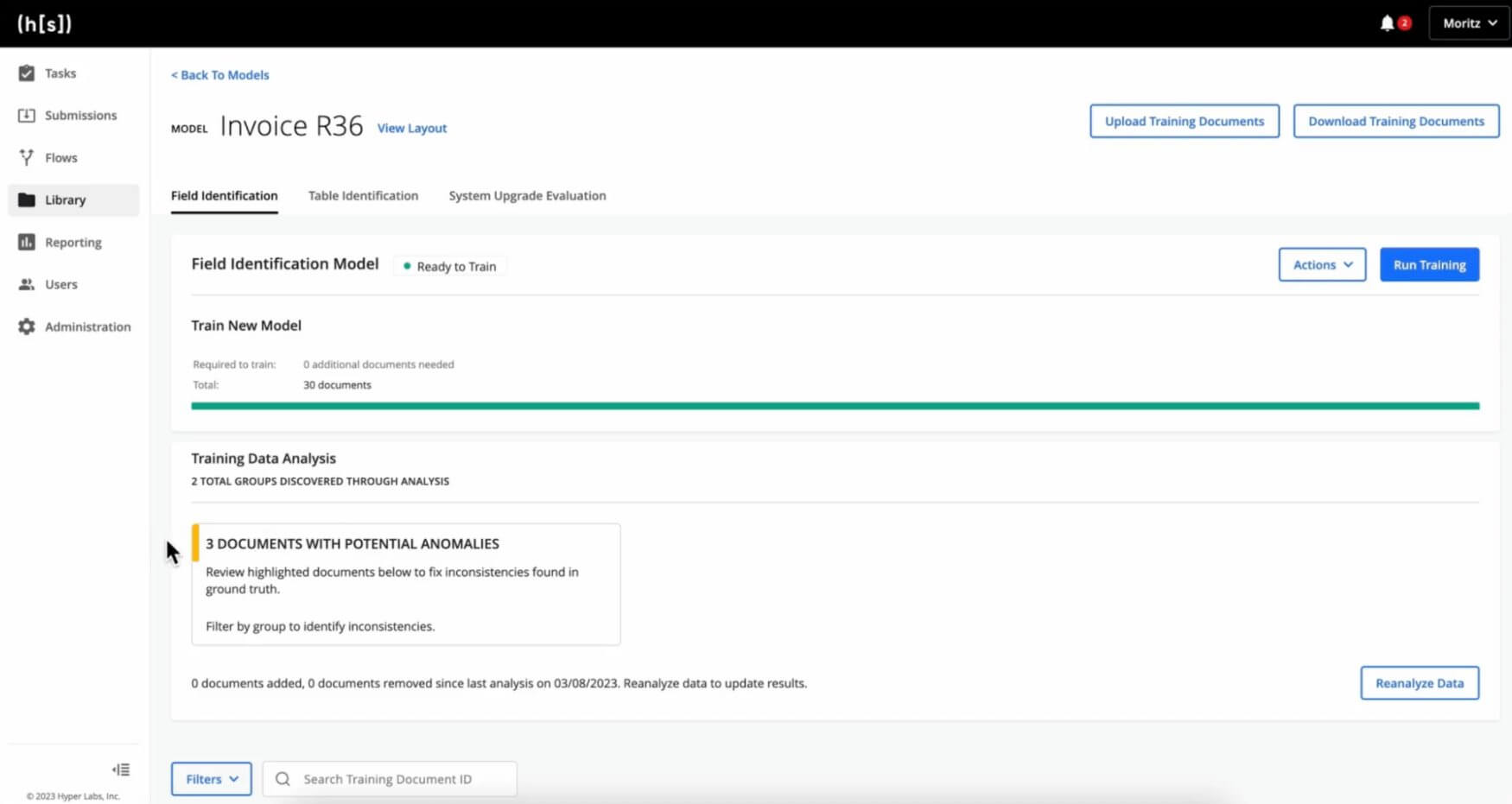
Step 3: Annotate the Documents
Only documents in the ‘Ready to annotate’ state can be annotated. For every document in your document set, identify the field you want the model to extract. After annotating a number of documents, the model will start suggesting annotations based on the previous documents you have already annotated.

Step 4: Run Data Analysis Again
After analyzing the data again, anomalies in your annotation are flagged with a yellow highlight. We recommend sorting documents by group or filtering by clusters to easily identify documents with anomalies.
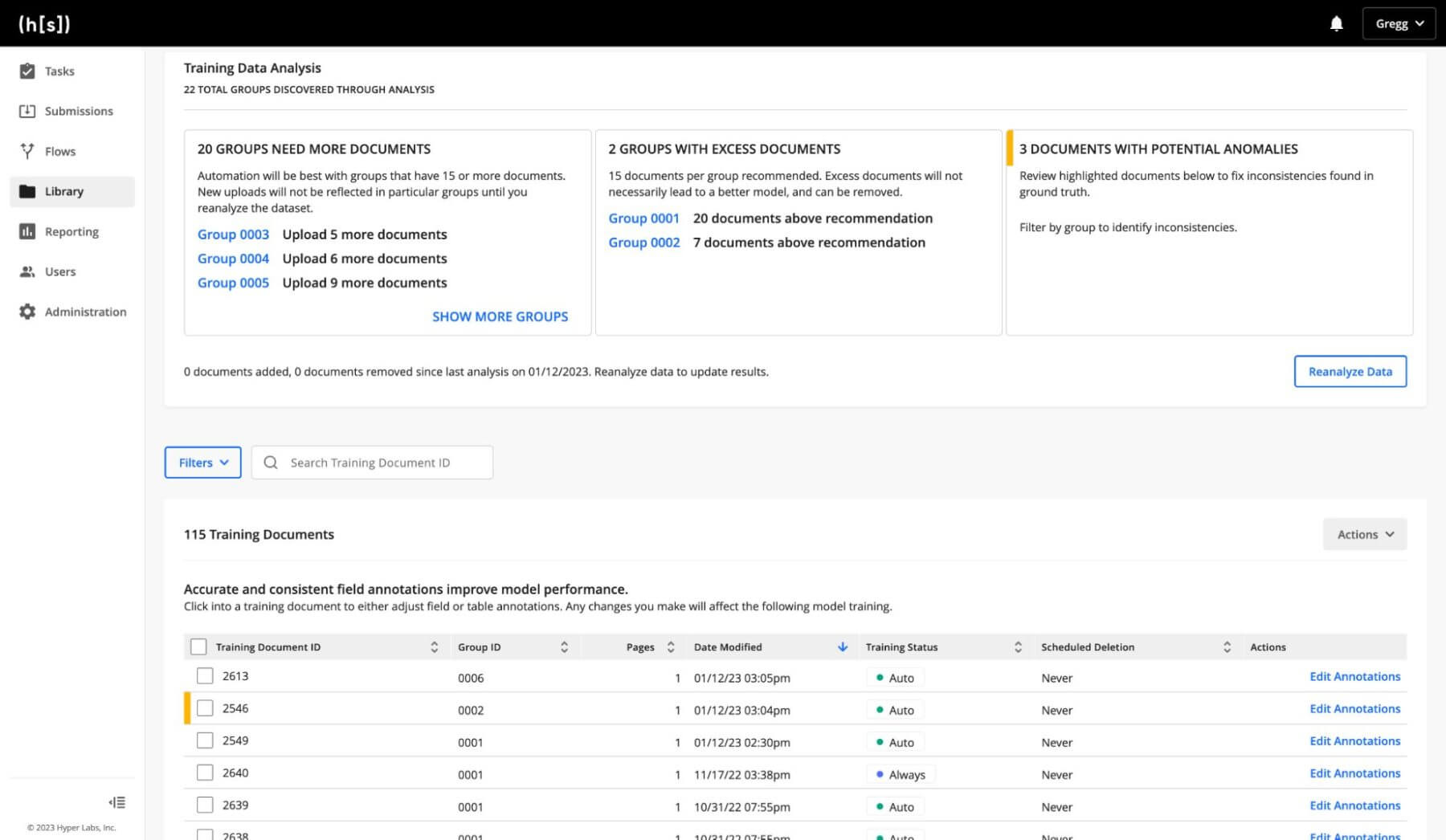
Step 5: Fix the Anomalies
Review the documents with possible anomalies identified, and either correct them or mark it as correct. In the Review Field Annotation section, you will be able to see how many anomalies exist in the document as well as which of the fields are anomalous. The erroneous annotation is surrounded by a dotted dashed line.
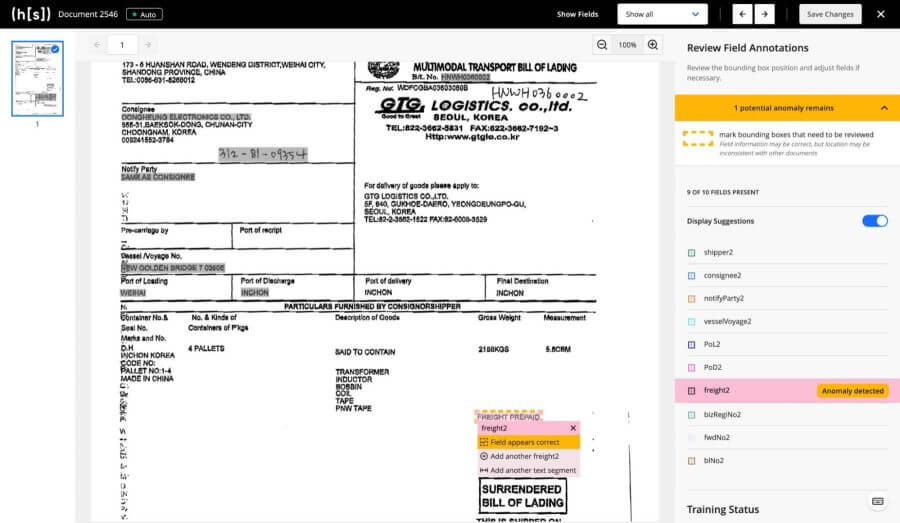
How to Get Started with Labeling Anomaly Detection
Labeling Anomaly Detection is available now in the R36 release of Hyperscience. To see a demo and learn more about how your business can benefit from Labeling Anomaly Detection, contact us here. For a closer look at this feature, you can read our technical documentation here.