In the dynamic world of AI and data processing, innovation and optimization drive technological progress. At Hyperscience, the Data Processing team embodies this commitment to advancement—an assembly of experts focused on refining user experiences, pushing boundaries, and leveraging data to enhance our platform’s capabilities.
Join us as we dive into the pivotal role this team plays, how their work improves our customer’s lives, and their exciting new process data showing an impressive 70% reduction in Field ID model training time.
The Data Processing Team’s Role
The Data Processing team is a vital part of Hyperscience’s Technical Services suite, primarily supporting Hyperscience’s customers and internal teams. Their core responsibilities include:
- Building and training models for Technical Validation Events (TVEs) and demos
- Providing support for active customers in production
- Performing Human-In-The-Loop services
- Resolving layout and model performance issues
- Conducting black box and exploratory testing of the platform
- Offering feedback on product issues and improvements
Essentially, they’re the power users of the Hyperscience Platform, familiar with tasks performed by regular users and more. Through working with complex use cases, they’ve become adept at building AI models swiftly and effectively. Beyond being AI model trainers, the team educates customers with best practices, expediting accurate model deployment. This expertise empowers customers to make the most of their data, diversify AI models, and build trust in the Hyperscience Platform.
The Team’s Experience in Action
To better understand the Data Processing team’s impact, let’s examine a recent project that assessed improvements in Hyperscience’s latest release.
The Project
The team compared model building and training between Hyperscience versions 35 and 37. They created two models in each version, semi-structured in type. For the use case, the team elected to use invoices, as they have Field ID and Table ID models, and have a rich variety in formats to create a complex dataset.
Both models used a shared dataset of 400 documents, and the project involved five components the Data Processing team follows in every project:
- Training data analysis
- Annotations
- Corrections of anomalies
- Model training
- Addressing MVts (model validation tasks)/anomalies
To accurately measure the changes, they tracked time spent on each phase.
The Results
Comparing time results, Field ID showed significant improvement: Total model building and training time in version 37 was reduced by 44.65% compared to version 35. The most notable enhancement occurred in the model training phase, where Field ID training time in version 37 was more than three times faster, thanks to speed optimizations built into the model training pipeline. As a result of the new Anomaly Detection and Training Data Curator features, Human-in-the-Loop tasks also improved, with correction time in version 37 dropping nearly 63% compared to version 35.
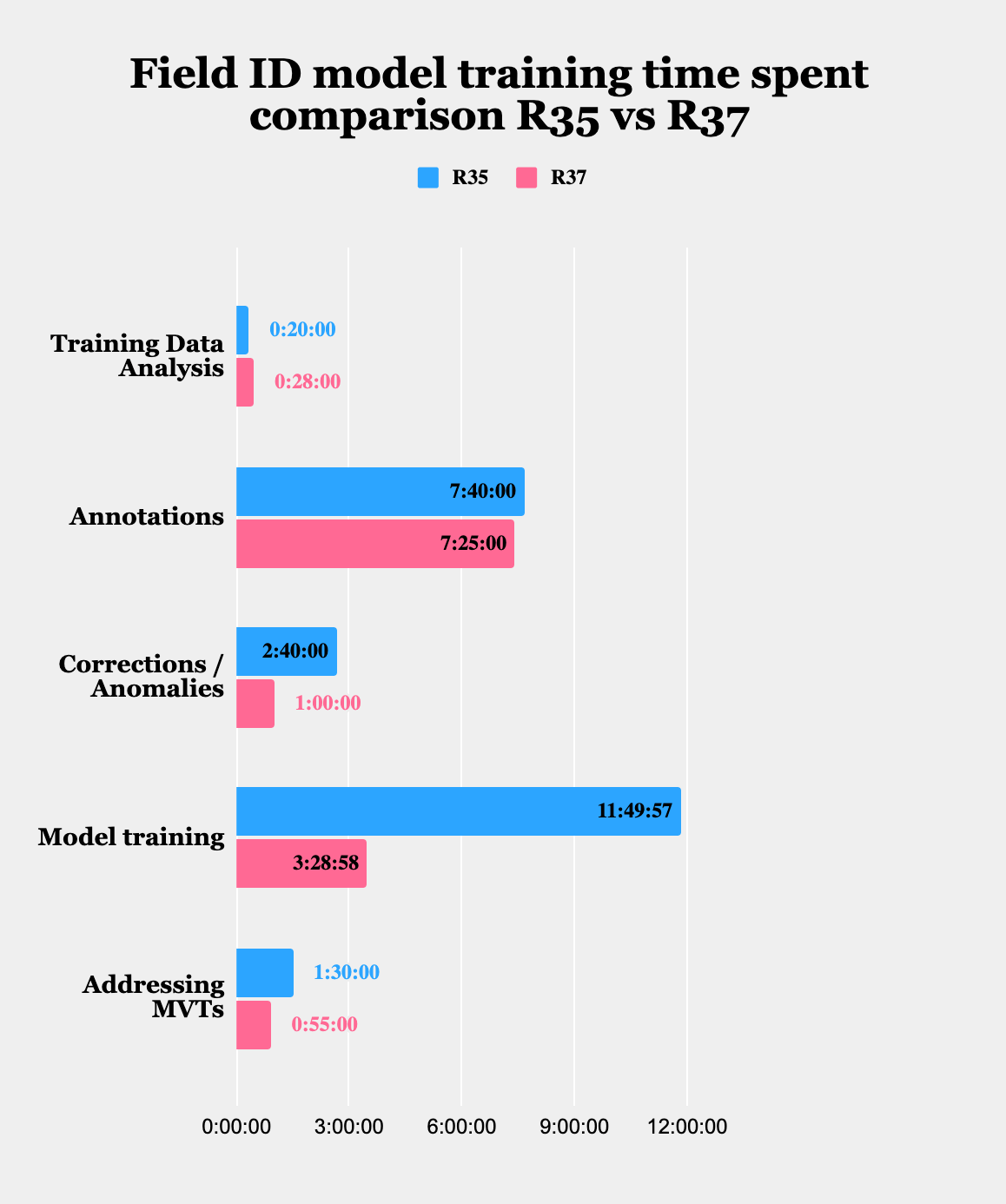
The Data Processing Team’s testing showed many improvements in Field ID Model Training times when compared to Hyperscience V35.
Additional Improvements
In their model-building process, the Data Processing team conducts a second round of checks across the dataset to identify inconsistencies and errors. Version 37 simplifies this with the Anomaly Detection feature, which accelerates error identification, contributes to a robust dataset, and creates a more efficient model.
Handling MVTs also improved. Version 37 integrates MVTs into the Anomaly Detection feature, expediting their resolution and giving users more control over task impact on model performance.
What Does this Mean for Hyperscience Users?
The Data Processing team’s work goes beyond data processing—it’s about saving time, enhancing efficiency, and user experiences. Through data-driven enhancements, they’ve showcased substantial improvements in model building and training.
And our commitment to innovation doesn’t stop with these improvements. We’re working to extend version 37’s improvements to other models, such as Table ID, to ensure enhanced model training benefits every user.
To unlock the time-saving advantages of Hyperscience version 37, reach out to your Customer Success Manager or Implementation Manager. There are many enhancements and improvements to benefit from—like streamlined model training and enhanced workflows—even without working directly with our Data Processing team. And by using the support of the Data Processing team to the fullest, the possibilities expand even further. With their assistance, you can not only maximize your Hyperscience proficiency, but also achieve greater levels of efficiency and effectiveness.